[ad_1]
Summary: Researchers pioneered the use of spatial omics and deep learning to craft 3D molecular maps of the brain, offering new insights into its function across different scales. Their study employs advanced mass spectrometry imaging and single-cell metabolomics to decode the brain’s intricate biochemistry.
This groundbreaking work aims to unravel the complex chemical interactions within the brain, potentially paving the way for breakthroughs in treating neurological diseases. The collaborative effort underscores the importance of interdisciplinary research in advancing our understanding of the brain’s molecular landscape.
Key Facts:
- Advanced Imaging Techniques: The team’s use of mass spectrometry imaging and single-cell metabolomics allows for high-resolution data collection and analysis of individual molecules in brain cells, marking a significant advancement in neuroscience research.
- Insight into Neurological Diseases: By creating detailed 3D maps that reveal the chemical distributions within the brain, the researchers aim to better understand neurochemical imbalances associated with diseases like depression and Alzheimer’s.
- Interdisciplinary Collaboration: The success of this research highlights the Beckman Institute’s collaborative culture, where cross-disciplinary interactions fuel innovative studies in brain science.
Source: Beckman Institute
Beckman Institute for Advanced Science and Technology researchers Jonathan Sweedler, a professor of chemistry, and Fan Lam, a professor of bioengineering, outlined how spatial omics technologies can reveal the molecular intricacy of the brain at different scales.
Their research appeared this month in Nature Methods.
The researchers and their colleagues used a biochemical imaging framework integrated with deep learning to create 3D molecular maps with cell specificity to better understand how the brain functions in health and disease. Their research is supported by a $3 million grant from the National Institute on Aging of the National Institutes of Health.
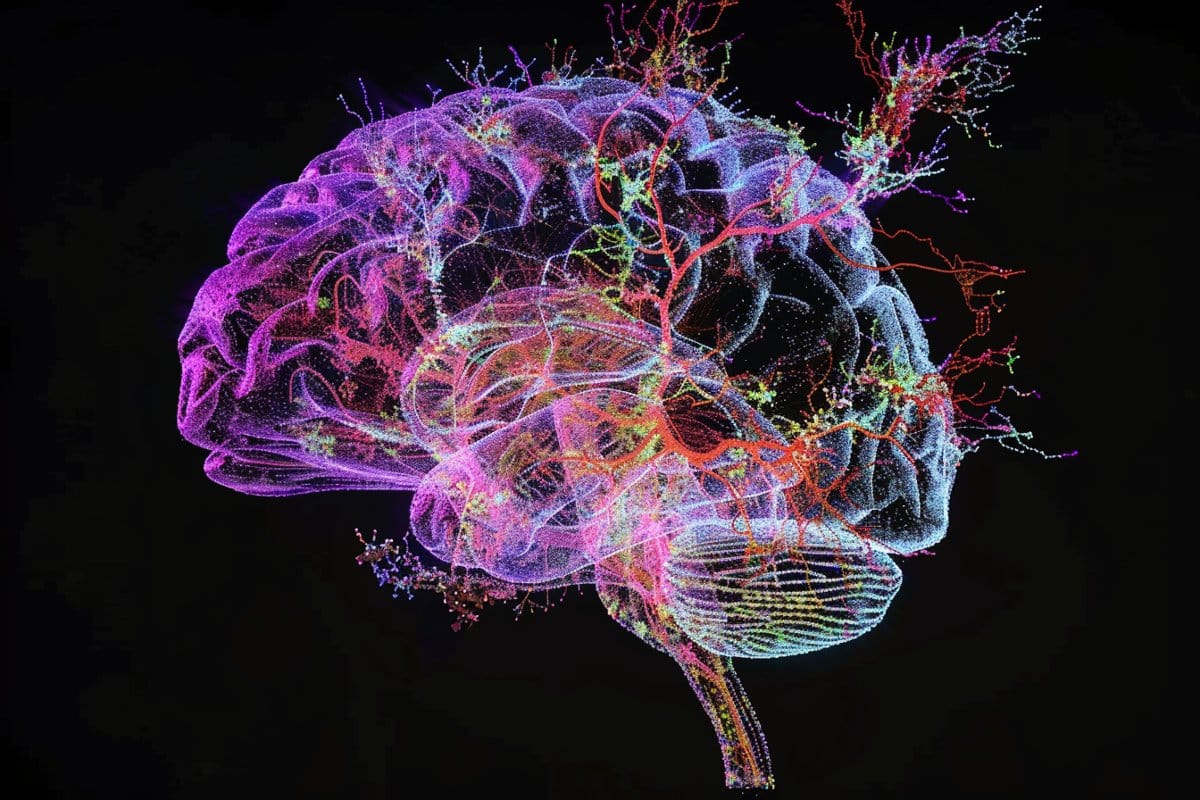
“If you look at the brain chemically, it’s like a soup with a bunch of ingredients,” Lam said. “Understanding the biochemistry of the brain, how it organizes spatiotemporally, and how those chemical reactions support computing is critical to having a better idea of how the brain functions in health as well as during disease.”
To understand how the brain’s chemical ingredients interact with one another, the researchers used a new imaging technique called mass spectrometry imaging to collect and analyze massive amounts of high-resolution data.
They also used single-cell metabolomics and computational tools to extract data about individual molecules in single brain cells, which enabled data acquisition at unprecedented speeds and scales.
“Most people have a feeling that brain diseases such as depression and Alzheimer’s are caused by neurochemical imbalances,” Sweedler said. “But those imbalances are really hard to study and it’s difficult to understand how chemicals interact at different scales (for example, at the tissue level and individual cell level) during problems in the brain.”
According to Sweedler, creating 3D maps of chemical distributions with cell-type specificity enables researchers to further understand the complicated biochemistry within the brain, which in the long term should help address currently intractable neurological diseases.
Single-cell metabolomics, a technology critical to the researchers’ findings, was named as one of Nature’s “Seven technologies to watch in 2023” along with CRISPR and the James Webb Space Telescope, speaking to the high impact these tools will continue to have as it relates to looking at cell-specific data, Sweedler said.
The research wouldn’t have been possible without the collaborative nature of the Beckman Institute.
“It truly amazes me how small interactions can turn into interesting research conversations and eventually into large-scale collaborative studies,” said first author Richard Xie, a Beckman Institute Graduate Fellow.
“The key is to be open-minded and interdisciplinary, as you may draw inspirations from another field. I feel very excited about the progress on leveraging different expertise across groups to engineer tools to better depict the biochemical landscape of the brain.”
Lam and Sweedler met at Xie’s behest to discuss his work on single-cell and tissue mass spectrometry imaging. The team had a breakthrough in how informatics and computational methods could lead to a new kind of multimodal, multiscale biochemical imaging that’s highlighted in their recent Nature Methods paper.
Funding: Research reported in this press release was supported by the National Institute on Aging of the National Institutes of Health under award number R01AG078797. The content is solely the responsibility of the authors and does not necessarily represent the official views of the National Institutes of Health.
About this AI and brain mapping research news
Author: Jenna Kurtzweil
Source: Beckman Institute
Contact: Jenna Kurtzweil – Beckman Institute
Image: The image is credited to Neuroscience News
Original Research: Open access.
“Multiscale biochemical mapping of the brain through deep-learning-enhanced high-throughput mass spectrometry” by Fan Lam et al. Nature Methods
Abstract
Multiscale biochemical mapping of the brain through deep-learning-enhanced high-throughput mass spectrometry
Spatial omics technologies can reveal the molecular intricacy of the brain. While mass spectrometry imaging (MSI) provides spatial localization of compounds, comprehensive biochemical profiling at a brain-wide scale in three dimensions by MSI with single-cell resolution has not been achieved.
We demonstrate complementary brain-wide and single-cell biochemical mapping using MEISTER, an integrative experimental and computational mass spectrometry (MS) framework.
Our framework integrates a deep-learning-based reconstruction that accelerates high-mass-resolving MS by 15-fold, multimodal registration creating three-dimensional (3D) molecular distributions and a data integration method fitting cell-specific mass spectra to 3D datasets.
We imaged detailed lipid profiles in tissues with millions of pixels and in large single-cell populations acquired from the rat brain. We identified region-specific lipid contents and cell-specific localizations of lipids depending on both cell subpopulations and anatomical origins of the cells.
Our workflow establishes a blueprint for future development of multiscale technologies for biochemical characterization of the brain.
[ad_2]
Source link