[ad_1]
Experimental framework
Parkinson’s disease patients (n = 4) undergoing DBS surgery performed the ultimatum game while we obtained electrochemical estimates of dopamine and serotonin in the SNr (Fig. 1a). Our electrochemistry protocol, which builds on earlier work in both animals34,35 and humans22,23,24,25,26, provides ten samples per second. In brief, the protocol involves the repeated delivery of a rapid change in electrical potential to a carbon-fibre electrode and measurement of induced electrochemical reactions as changes in current at the electrode tip. The current responses carry information about not only the identity but also the concentration of neuromodulators in the surrounding neural tissue. This information is extracted using a signal prediction model trained on large wet-lab datasets where the chemical environment can be carefully controlled (see Supplementary Fig. 1 for an illustration of the electrochemical approach as well as an in vitro evaluation of the signal prediction model; see Methods for details).
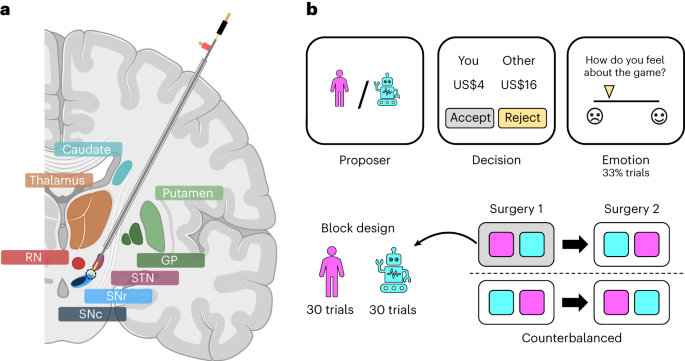
a, Illustration of electrode trajectory and recording site. GP, globus pallidus; STN, subthalamic nucleus; SNr/SNc, substantia nigra pars reticulata/compacta; RN, raphe nucleus. Brain slice created with Biorender.com. b, The game involved 60 trials of the one-shot ultimatum game where participants had to accept or reject splits of a US$20 stake proposed by either a human (30 trials) or a computer (30 trials) avatar. On around a third of trials, participants were asked to indicate how they felt about the game. Each participant underwent two surgical sessions 14–28 days apart; the human and computer conditions were blocked within a session and their order was counterbalanced across sessions.
The clinical treatment involved two surgeries 14–28 days apart for the bilateral implantation of DBS electrodes in the subthalamic nucleus of each hemisphere, and participants performed the ultimatum game in each surgical session (4 patients × 2 sessions = 8 datasets). Within a session, participants played the role of the responder in a series of one-shot interactions with human or computer avatars (30 trials × 2 conditions = 60 trials; Fig. 1b). The human avatars were unique, with each avatar having its own name and visual image, whereas the computer avatar was the same on each trial. The two conditions were blocked within a session and counterbalanced across sessions. In each condition, participants were asked to accept or reject splits of a US$20 stake. The human and computer avatars were both programmed to make offers between US$1 and US$9, with the same set of offers used in each condition in a session but in a randomized order. Participants were not given this information. On around a third of trials, participants were asked to rate how they felt about the game by moving a slider along a visual analogue mood scale ranging from negative (sad emoji) to positive (happy emoji).
Behavioural results
To unpack participants’ task behaviour, we first ran a logistic mixed-effects model in which we predicted choices (reject = 0, accept = 1) using offer value, condition (computer = −0.5, human = 0.5), and the interaction between these terms (choice model 1 (C-M1); see Methods for details about the statistical analysis of behavioural and neural data which was conducted at the trial level). As expected, value had a positive effect on choice (positive slopes in Fig. 2a; C-M1, value, t(454) = 2.43, P = 0.016, β ± 95% confidence interval (CI) = 1.77 ± 1.43): participants accepted 43% of offers smaller than, or equal to, one standard deviation (US$2) below the mean (US$5), 58% of offers within one standard deviation of the mean, and 94% of offers equal to, or larger than, one standard deviation above the mean. In keeping with earlier results30,31,32,33, condition had a negative effect on choice (pink below cyan in Fig. 2a; C-M1, condition, t(454) = −2.23, P = 0.026, β ± 95% CI = −3.60 ± 3.18): participants accepted 50% of the offers made by human avatars but 75% of those made by the computer avatar. There was no interaction between value and condition (C-M1, value × condition, t(454) = 1.35, P = 0.176, β ± 95% CI = 1.70 ± 2.47).
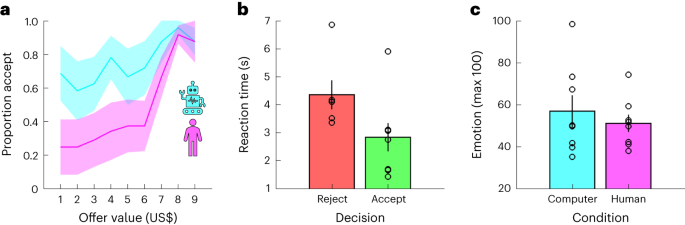
a, Proportion accept (y axis) given offer value (x axis) and condition (colours). b, Reaction time (y axis) given choice (colours). c, Emotion (y axis; 0–100) given condition (colours). b,c, Each dot is a dataset. a–c, Data are represented as mean ± s.e.m. across datasets (n = 8).
Previous studies have found that participants adapt their willingness to accept an offer to the history of offer values, including when offers are made by unique avatars and offer values are randomized36,37. To test for behavioural adaptation, we re-ran the logistic mixed-effects model after adding the difference in value between the current and the previous offer—akin to a one-step RPE—as a predictor (C-M2). The effects of value and condition remained (C-M2; value, t(423) = 2.82, P = 0.005, β ± 95% CI = 1.79 ± 1.25; condition, t(423) = −2.38; P = 0.018, β ± 95% CI = −3.67 ± 3.03; value × condition, t(423) = 0.90, P = 0.371, β ± 95% CI = 1.13 ± 2.47), but there were no history effects (C-M2; value difference, t(423) = 0.38, P = 0.707, β ± CI = 0.12 ± 0.61; value difference × condition, t(423) = 0.59, P = 0.558, β ± 95% CI = 0.47 ± 1.57). Indicative of some behavioural adaptation being at play, the model that included history effects provided a better fit to the data than the basic model (Akaike information criterion (AIC), C-M1 = 3016, C-M2 = 2,843). We note that the absence of an interaction between value difference and condition indicates that the use of a single avatar (computer) versus multiple avatars (human) did not affect task behaviour.
We next used linear mixed-effects models to analyse reaction times (RT model 1 (RT-M1)) and the emotion ratings (emotion model 1 (E-M1)). In addition to the terms from the choice analysis (C-M1), we included choice (reject = −0.5, accept = 0.5) and the interactions between choice and the other terms. There was an effect of choice on reaction times, with participants taking longer to reject than accept an offer (Fig. 2b; RT-M1, choice, t(450) = −2.56, P = 0.011, β ± 95% CI = −0.37 ± 0.28). However, there were no effects of value or condition on reaction times (RT-M1, all absolute t(450) < 1.62, all P > 0.106). In addition, the analysis of the emotion ratings did not return any effects (Fig. 2c; E-M1, all absolute t(147) < 1.70, all P > 0.093).
Overall dopamine tracks social context
Having characterized participants’ task behaviour, we turned to the electrochemical data to test whether dopamine and serotonin tracked the social context and value signals embedded in our task. Considering the results obtained from pharmacological manipulations of dopamine and serotonin levels during economic games10,11,14,30, we first asked whether overall levels of dopamine/serotonin varied with the decision to accept or reject an offer and the social context. To this end, we ran a linear mixed-effects model in which we predicted trial-by-trial estimates of overall levels of dopamine/serotonin using choice, condition and their interaction (neural model 1 (N-M1)). Here, overall dopamine/serotonin levels were defined as the sum of samples within a 1 s window after offer presentation.
This analysis indicated that overall levels of dopamine, but not serotonin, were modulated by social context. Specifically, while there were no choice-related effects on dopamine, there was a positive effect of condition, with higher dopamine in the human than the computer condition (pink above cyan in Fig. 3a, top; N-M1; choice, t(454) = −0.29, P = 0.771, β ± 95% CI = −0.03 ± 0.20; condition, t(454) = 3.04, P = 0.002, β ± 95% CI = 0.85 ± 0.55; choice × condition, t(454) = −0.69, P = 0.493, β ± 95% CI = −0.17 ± 0.50). In other words, while dopamine may drive a general change in the willingness to accept an offer made by a human versus a computer (Fig. 2a), it does not drive individual choices per se. In contrast, there were no effects on serotonin (Fig. 3a, bottom; N-M1; choice, t(454) = −0.57, P = 0.570, β ± 95% CI = −0.06 ± 0.21; condition, t(454) = −0.01, P = 0.993, β ± 95% CI = 0.00 ± 0.58; choice × condition, t(454) = −1.21, P = 0.227, β ± 95% CI = −0.32 ± 0.53).
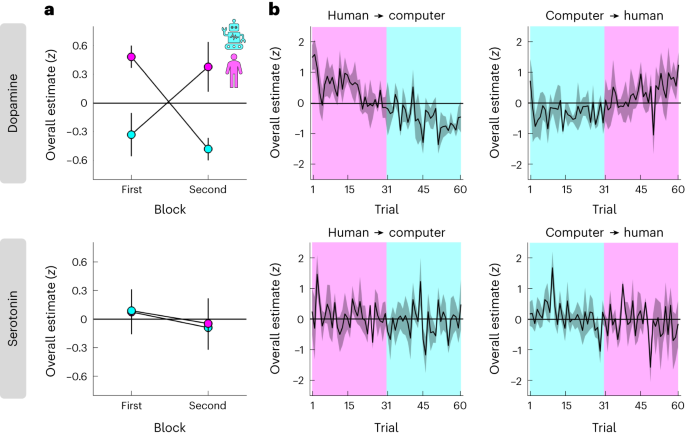
a, Overall dopamine/serotonin (y axis) separated by condition (colour) and its order within a session (x axis). b, Overall dopamine/serotonin (y axis) across time separated by condition order (left column versus right column). a,b, Overall estimates were computed as the sum of neuromodulator samples within a 1 s window (ten samples) after offer presentation. We limited the estimates to this window for consistency with the relative analysis in Fig. 4 and to ensure that all estimates were based on the same number of samples regardless of variation in reaction times and trial events (for example, variable duration of proposer screen and emotion ratings). The effect of condition on dopamine remained regardless of the specific time window (for example, a 6 s window centred on offer presentation; N-M1, t(454) = 2.63, P = 0.009, β ± 95% CI = 1.00 ± 0.75). Data are represented as mean ± s.e.m. across datasets (n = 4 for each order). Each dataset (session) was z-scored separately.
Our counterbalanced block design (Fig. 1b) allowed us to control for order effects such as changes in task engagement due to fatigue. To this end, we re-ran the linear mixed-effects model after including the order of a condition within a session (first = −0.5, second = 0.5) and its interactions with the other terms as predictors (N-M2). In further support of an interpretation that overall dopamine levels depend on social context, the analysis again identified a positive effect of condition, but no order-related effects, on dopamine (compare order in Fig. 3a, top; N-M2; condition, t(450) = 2.98, P = 0.003, β ± 95% CI = 0.85 ± 0.56; remaining effects, all absolute t(450) < 0.76, all P > 0.451). There were again no effects on serotonin (Fig. 3a, bottom; N-M2; all absolute t(450) < 1.37, all P > 0.172). Consistent with an absence of order-related effects, the model that included order effects provided a worse fit to the data compared to the basic model (AIC; dopamine, N-M1 = 1,205, N-M2 = 1,263; serotonin, N-M1 = 1,270, N-M2 = 1,331). Visualization of the full trial-by-trial data indicated that the effect of social context on overall dopamine levels reflected gradual changes across time (Fig. 3b).
Relative dopamine and serotonin track value signals
Having established an impact of social context on overall dopamine levels, we asked whether changes in dopamine/serotonin relative to a local baseline reflected value signals as predicted by both empirical and theoretical work15,16,17,18,19. To this end, we ran a linear mixed-effects model in which we predicted trial-by-trial estimates of relative changes in dopamine/serotonin using the value of the current offer and the difference in value between the current and the previous offer—akin to a one-step RPE (N-M3). Here, relative dopamine/serotonin levels were defined as the sum of samples within a 1 s window after offer presentation, but now after having first subtracted the sample at offer presentation as a local baseline.
This analysis indicated that relative changes in dopamine and serotonin reflected distinct yet complementary value signals. In support of a role in RPE signalling, value difference, but not value per se, had a positive effect on dopamine (Fig. 4, left column; N-M3; value, t(426) = −1.47, P = 0.144, β ± 95% CI = −0.10 ± 0.14; value difference, t(426) = 2.82, P = 0.005, β ± 95% CI = 0.21 ± 0.14): dopamine showed a relative decrease when the current offer was lower than the previous one (akin to a negative RPE) and a relative increase when the current offer was higher than the previous one (akin to a positive RPE). In contrast to this response pattern, value, but not value difference, had a positive effect on serotonin (Fig. 4, right column; N-M3; value, t(426) = 3.15, P = 0.002, β ± 95% CI = 0.22 ± 0.14; value difference, t(426) = −1.82, P = 0.070, β ± 95% CI = 0.13 ± 0.14): serotonin showed a relative increase for high offers and a relative decrease for low offers. To control for any model misestimation due to the correlation between value and value difference (Pearson’s r = 0.73), we regressed value difference against value and used the residuals (Pearson’s r ≈ 0) as our predictor for value difference (N-M3*). In keeping with the original results, this analysis returned an effect of value difference on dopamine (N-M3*; value, t(426) = 0.82, P = 0.412, β ± 95% CI = 0.05 ± 0.11; value difference, t(426) = 2.82, P = 0.005, β ± 95% CI = 0.21 ± 0.14) and an effect of value on serotonin (N-M3*; value, t(426) = 2.67, P = 0.008, β ± 95% CI = 0.13 ± 0.09; value difference, t(426) = −1.82, P = 0.070, β ± 95% CI = −0.13 ± 0.14).
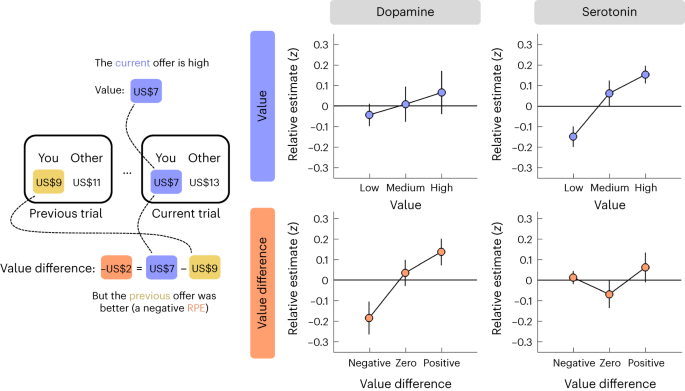
To visualize the value effects (see main text for statistical analysis), we grouped the value of the current offer (top row) and the difference in value between the current and the previous offer (bottom row) into three bins using terciles and then plotted relative dopamine/serotonin (y axis) for each bin (x axis). Relative estimates were computed by first subtracting the sample at offer presentation as a local baseline and then taking the sum of neuromodulator samples within a 1 s window (ten samples) after offer presentation. Data are represented as mean ± s.e.m. across datasets (n = 8). Each dataset (session) was z-scored separately.
Recent research suggests that serotonin tracks absolute (unsigned) RPEs, which provide an estimate of variability in the environment and can be used to regulate the rate of learning19,38. Since the visualization of relative changes in serotonin was consistent with this computational function (U shape in Fig. 4, bottom right), we tested this hypothesis formally by also including the absolute value difference as a predictor (N-M4). However, while this analysis replicated the original results, it did not identify an effect of absolute value difference for dopamine (NM-4; value, t(425) = −1.48, P = 0.139, β ± 95% CI = −0.11 ± 0.14; value difference, t(425) = 2.87, P = 0.004, β ± 95% CI = 0.21 ± 0.14; absolute value difference, t(425) = 0.92, P = 0.360, β ± 95% CI = 0.08 ± 0.17) or serotonin (N-M4; value, t(425) = 3.07, P = 0.002, β ± 95% CI = 0.22 ± 0.14; value difference, t(425) = −1.78, P = 0.076, β ± 95% CI = −0.13 ± 0.14; absolute value difference, t(426) = 0.18, P = 0.861, β ± 95% CI = 0.02 ± 0.15). In addition, the model itself provided a worse fit to the data compared to the basic model AIC; dopamine, N-M3 = 1,231, N-M4 = 1,243; serotonin, N-M3 = 1,231, N-M4 = 1,244).
Finally, we asked whether the value-related effects were modulated by social context. To this end, we re-ran the linear mixed-effects model after including condition and its interaction with the value-related terms as predictors (N-M5). In support of a hypothesis that relative changes in dopamine and serotonin reflect generalized value signals, the analysis replicated the earlier effects, but did not identify any condition-related effects, for dopamine (N-M5; value, t(423) = −1.36, P = 0.176, β ± 95% CI = −0.11 ± 0.16; value difference, t(423) = 2.63, P = 0.009, β ± 95% CI = 0.21 ± 0.15; condition-related effects, all absolute t(423) < 0.93, all P > 0.354) or serotonin (N-M5; value, t(423) = 3.00, P = 0.003, β ± 95% CI = 0.21 ± 0.14; value difference, t(423) = −1.72, P = 0.086, β ± 95% CI = −0.12 ± 0.14; condition-related effects, all absolute t(423) < 0.86, all P > 0.390). In line with an absence of modulation by social context, the model itself provided a worse fit to the data compared to the basic model (AIC; dopamine, N-M3 = 1,231, N-M5 = 1,262; serotonin, N-M3 = 1,231, N-M5 = 1,273).
[ad_2]
Source link